Best Practices: Setting the New Standard for Investment Data Accuracy
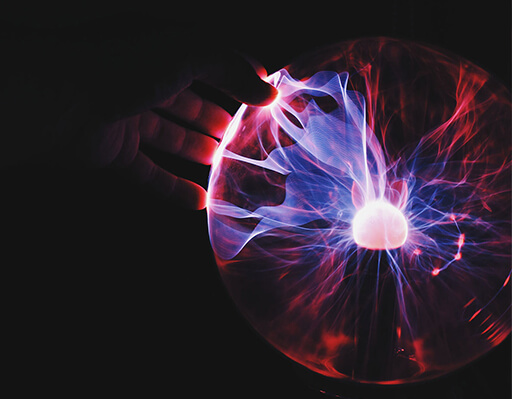
Introduction
Since we founded Canoe Intelligence, we’ve worked tirelessly to build technology that makes the collection and validation of alternative investment reporting data more accurate and efficient. To us, this means a 100% accuracy rate in terms of ingesting, categorizing, extracting, and validating these critical data inputs.
But, that doesn’t necessarily mean this process will be 100% automated (even with the most cutting-edge technology in the world). At best, we hope to achieve 99% processing success rates. The reality is, there are changing formats, poor-quality images, and complexities within the documents for which your team’s expertise is needed. As an investment or operations professional, you know your clients and their investments like the back of your hand, and your institutional knowledge is invaluable.
In building robust exception reporting, our goal is to make it easier for professionals like you to allocate your time toward the documents that require your attention, while straight through processing everything else with 100% accuracy.
In this article, we walk you through the process that our team has developed to make 100% data accuracy a reality for our clients.
Already intrigued by what you have read? Schedule a demo.
How Does the System Learn?
Canoe has built a collective intelligence across millions of documents over more than six years. Each document contributes to an ever-growing intelligence that gets applied to new documents in order to process and extract relevant data points. As new documents are processed in the technology, learning patterns are created and stored for the shared benefit of clients.
A learning pattern can be created with as little as one document; however, we typically see four to six documents as the sweet spot for robust, reliable learning pattern creation. Once a learning pattern is established, it is utilized in subsequent months or quarters. With each learning pattern created, Canoe is expanding a foundation from which to extract relevant data elements in sub-seconds.
But, what happens if the technology is not 100% confident in a certain document or data point? In most machine-learning-based technologies, the systems will “guess” and provide the user with a confidence level. At scale, this is not a realistic approach. Would you trust a technology that is telling you it is 75% confident that the Ending Capital Balance is $1,500,000? Absolutely not.
Our approach is far more suitable for real-world workflows. We have infused the technology with accounting and investment rules to flag potential data exceptions. Moreover, we’ve rid the system of the dreaded “confidence level.” For example, if Canoe is not 100% confident that the Ending Capital Balance is $1,500,000, then you will receive an Exception Flag, and can personally check or resolve the data point in question. With Canoe, 90 to 95% of documents are systematically processed in sub-seconds, and your expertise will positively enhance the intelligence and accuracy for the remaining 5-10% of documents, depending on document type and complexity.
And, this is where our clients become very engaged in this process. In the below example, the technology proactively flagged the month-to-date return figure, as it does not match the calculated expectation. You would receive a notification for this exception, and can then review and take action immediately in the system.

No longer do you have to sift through hundreds of thousands of documents looking for the one potential data issue. By proactively flagging exceptions on data points and elements of importance, or highlighting documents that need additional configuration, you can review the smaller selection of documents and personally validate the data. It’s important to us that the system receive this validation from our clients instead of guessing, so we can ensure 100% accuracy going forward. An added benefit is that clients tell us they’re fresher when reviewing the complex documents that actually require their attention as they haven’t spent all day mindlessly categorizing documents and extracting data from them!
Have more questions about how the system learns? Schedule a time to go over it with us.
Flexibility is Key
We know that every administrator reports data in different formats. This can be challenging for traditional data capture tools. Based on intelligence created from millions of documents, Canoe’s technology is able to go beyond basic extraction tools and can identify specific tables, text formats, and more to understand how each fits into the construct of the document and associate a pattern from the collective intelligence. So, if a sponsor changes administrators, there’s a likelihood that Canoe will have some ability to recognize the new format based on a history of patterns created across similarly formatted documents. This flexibility adds to our ability to identify and validate vital data elements even when the actual reports may shift in appearance.
Moreover, we’ve been deliberate about building a proprietary technology that blends best-in-class data capture tools. Combining NLP, text anchoring, spatial recognition, and coordinate mapping, Canoe analyzes the metadata layered within a document, resulting in the most effective and flexible data extraction technology available. Unlike traditional OCR technologies that break when a format changes or table moves, Canoe’s technology accommodates ever-changing formats. And again, where the system lacks 100% confidence, our users know about it immediately.
The New Standard
By partnering with our clients to build Canoe Intelligence in a way that prioritizes learning, accuracy, and flexibility, we are actively setting the new standard for success in data entry for our industry.
Canoe Intelligence is the first and only technology that automates the highly frustrating, time-consuming, and costly manual workflows related to document management and data extraction for alternative investment reporting. Schedule a demo with us today to learn more.